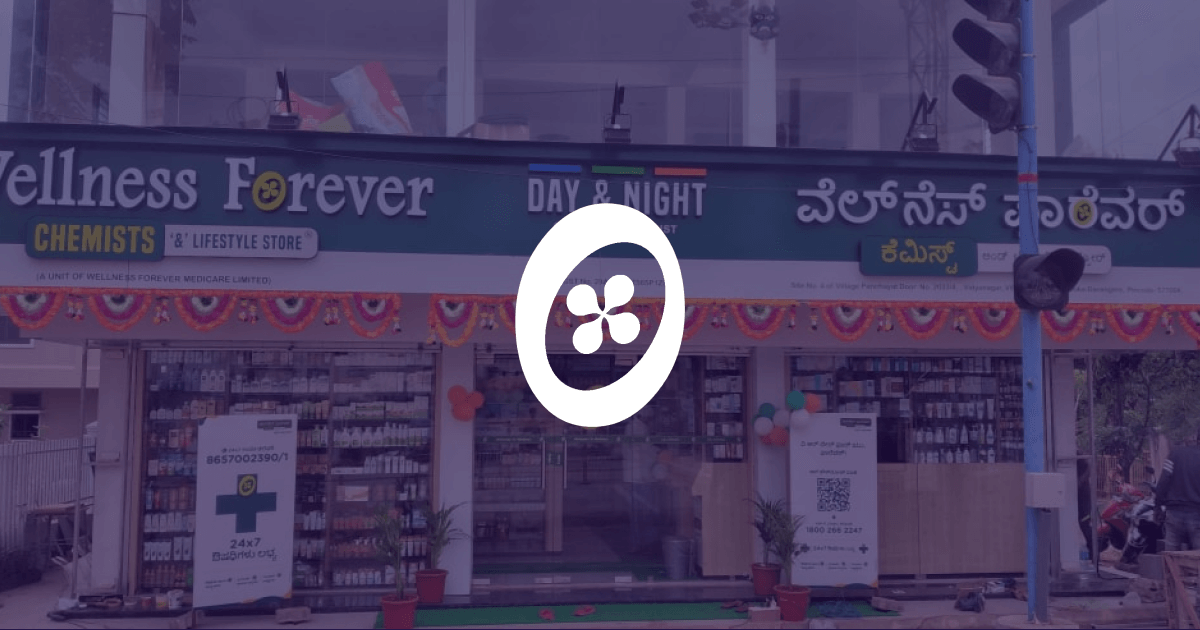
Utilizing AppsFlyer revenue recommendations to optimize SKAN campaigns and drive ROI
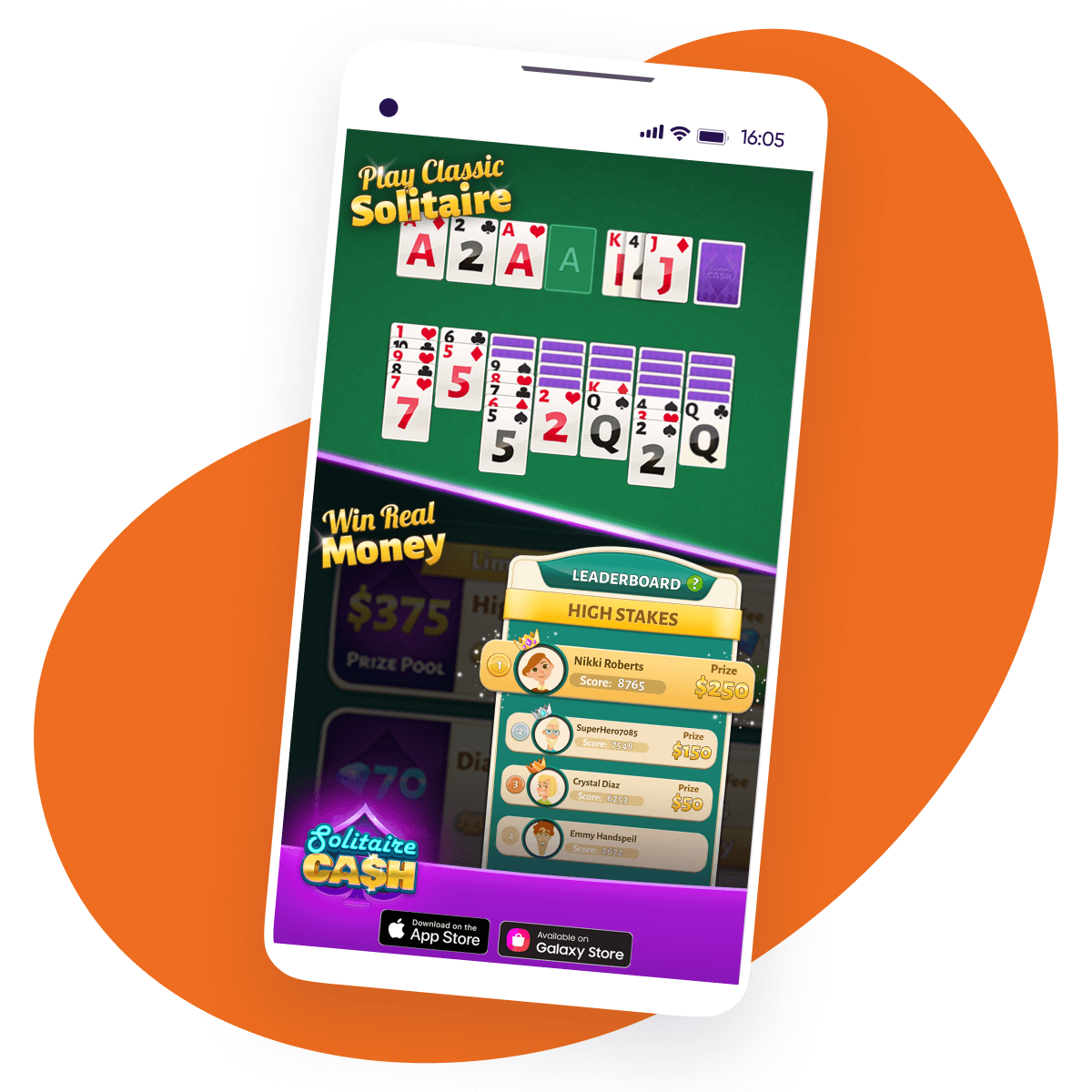
114%
Increase in ROI83%
Decrease in CPIBackground
Papaya Gaming is a fast-growing mobile gaming company. Its main specialty is creating skill-based, real money popular casual games such as Solitaire and Bubbles. The company’s secret sauce is its “matchmaking” technology that enables players with a similar level of skills to compete against each other. Papaya’s games have been downloaded over 17 million times worldwide and have an impressive average rating of 4.7/5 in the App Store.
Challenge
Before Apple’s privacy changes were announced, Papaya’s main focus for growth was the iOS user-base; its channels of choice for mobile ads were self-reporting networks (SRNs). “We were mainly focused on SRNs for mobile advertising,” says Uri Pearl, Papaya’s Head of Marketing. “It was what worked for us at the time.”
iOS 14 forced Papaya to rethink its mobile marketing strategy: “At first, the news felt apocalyptic. We had to figure out how we could still optimize and measure our campaigns effectively in the new reality,” Uri recalls. “We were on top of the iOS changes from the get-go, and realized that accurate SKAdNetwork reporting on installs and post-install activity was going to be critical for our success.”
Papaya also began diversifying their marketing channels and allocating part of their budget to non-SRN ad networks, while continuing to maintain and optimize SKAN-based campaigns.
“SKAdNetwork was a learning curve,” agrees Dan Hayoun, Papaya’s UA Team Lead. “Initially we were having difficulties optimizing our campaigns, our CPI numbers were through the roof.” Papaya was also experiencing an extremely high percentage of nulled conversion values, making the task of optimizing conversion values incredibly challenging.
Solution
At first, Papaya took to manually updating its apps’ conversion value schemas, testing and adjusting to optimize results; not long after, it started using AppsFlyer’s Conversion Studio. “With Conversion Studio, we were able to make changes to our conversion value schema in just a few clicks – without dealing with all of the technicalities,” Dan recalls. “That made testing different schemas much easier”.
Although the company was able to configure its conversion value schemas on the fly and perform multiple tests, Papaya was far from reaching its goals. “We had an ROI that was significantly lower than what we had prior to iOS 14, and our average CPI was 10x higher!,” Uri says. “We started using the custom mode in Conversion Studio, and soon after AppsFlyer reached out and asked if we wanted to test a new feature that provides conversion value revenue recommendations based on your app’s data.”
The new feature is called Revenue Recommendations. Revenue Recommendations ensure conversion value schemas are optimized for revenue measurement. In order to provide recommendations, AppsFlyer operates an algorithm that scans the app’s LTV data to learn more about users’ spending habits and how revenue is typically distributed.
To illustrate this with a simple use case, let’s imagine an app measures revenue in $100 ranges (0-100, 100-200, etc). However, the AppsFlyer algorithm detects that most users spend $0-$10, and there are also some users that spend $100-$120.
Because the revenue recorded is the average of the range, users who spend $10 will be recorded as spending $50 and users who spend $100 will be reported as spending $150. Needless to say, that doesn’t reflect reality. In this case, AppsFlyer would recommend a new schema that is more representative of users’ spending habits.
Papaya selected a single app to try out the new feature. “AppsFlyer analyzed our data and returned with a set of revenue recommendations. The recommendations we got were based on thousands of our installs and in-app revenue data, and were backed up by graphs featuring revenue distribution and outliers,” says Uri. “We were able to see the margin of error and the effect each recommendation would have on our data.”
Results
“The impact was immediate. As soon as we started using Revenue Recommendations our ROI increased by 114% and our CPI dropped by 83%. Beforehand, I was unable to run a campaign for more than a few days, now I have sustainable campaigns that run for months on end.”
Uri Pearl, Head of Marketing
Changing the conversion value schemas also enabled Papaya to measure more with less. “By decreasing our revenue ranges and getting rid of outliers, we can measure more with fewer bits. In the past, we needed all 63 values to measure a specific revenue range, now we’re using 30 to measure the entire user LTV,” says Dan.
Using 30 values instead of 63 means Papaya is also able to devote 1 bit to Single Source of Truth (SSOT) — AppsFlyer’s solution to dedupe multiple attribution data streams for a complete and accurate picture of performance — and dedicate the rest toward measuring in-app events.
Looking ahead
When Papaya began using Revenue Recommendations, it only tested one app. Papaya has since expanded its use of Revenue Recommendations to all of its iOS apps. “AppsFlyer’s continuous release of new features enables us to do more with our own data,” says Uri. With new apps in development and a broader focus on both iOS and Android, the year ahead holds exciting things for this rapidly growing company.