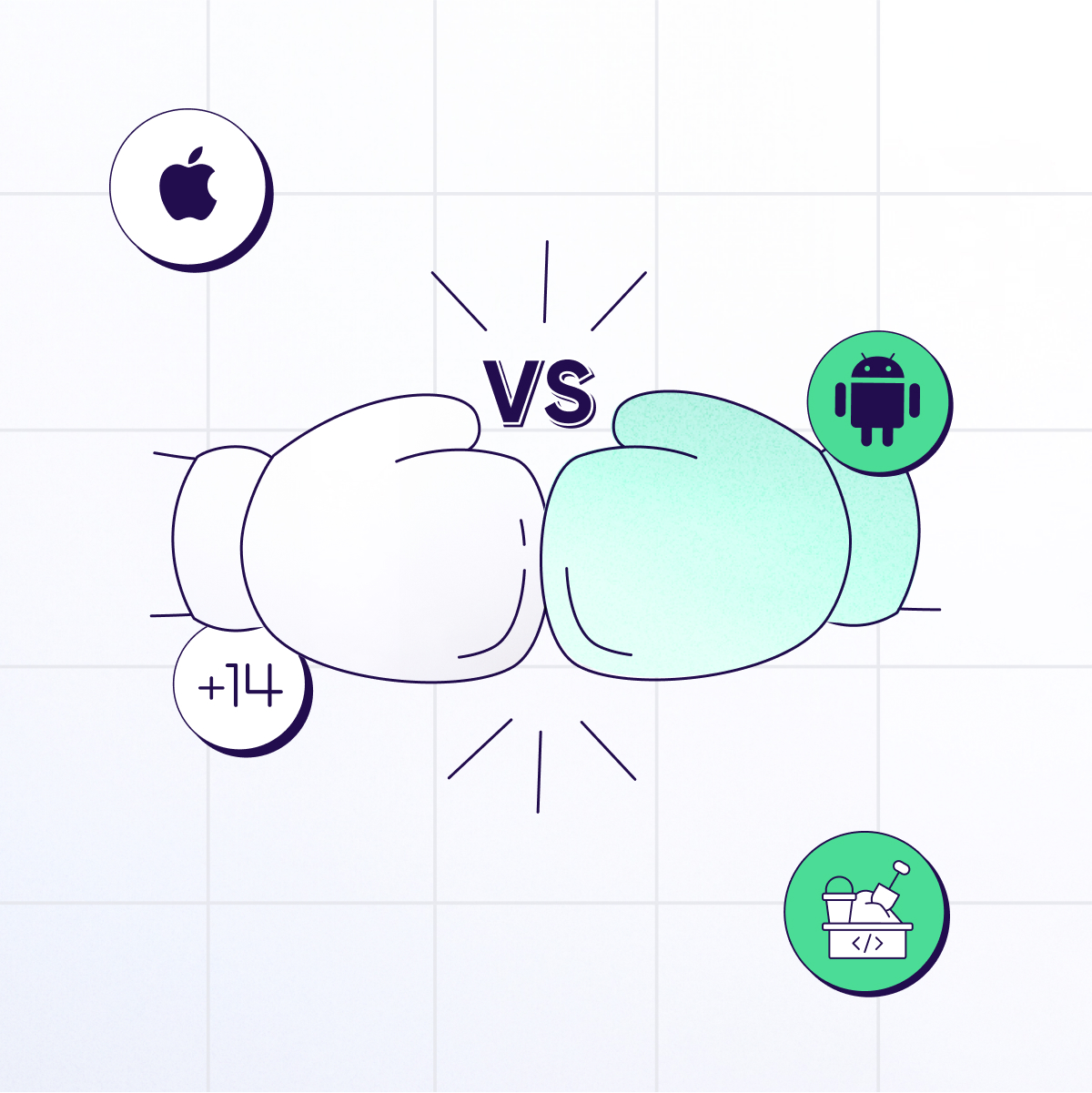
How to overcome signal loss by building a new data reality
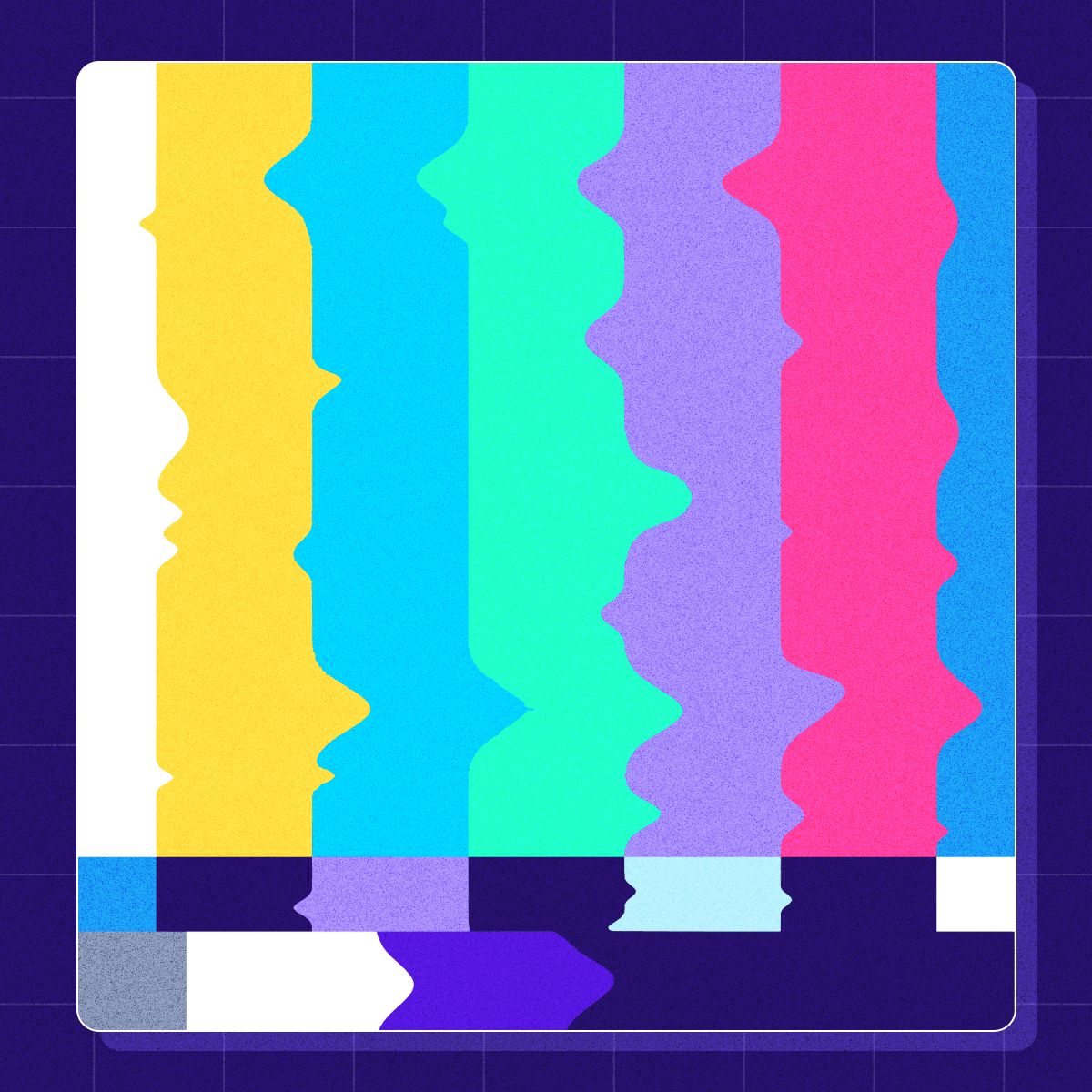
The privacy era has led to a loss of data signals that has significantly hampered marketers’ ability to target, measure, and optimize their campaigns. According to Mckinsey, up to $10 billion is at risk because of signal loss — in the US alone!
But thanks to innovation and adaptation, overcoming the challenge is more than possible by building a new data reality based on filling gaps in existing data, as well as creating new data signals.
In this blog, we’ll explain what led to this reality, the impact of signal loss on marketing, and how to overcome it. Let’s dive in!
What is signal loss
The loss in data signals as the result of diminishing levels of user level data identifiers. These identifiers have been, and in some cases still are, the backbone on which performance-driven marketing measurement is based on. Signal loss is therefore a massive change and challenge in the digital marketing landscape.
Background: What led to this reality
In recent years, there’s been increased scrutiny from legislators and consumers to protect user privacy through various regulations (GDPR, CCPA, DMA, to name a few major recent ones).
At its core, it’s about controlling how user level data is being shared across different companies and how consent to share this data is requested and presented.
In parallel (whether reactive or proactive), Google and Apple have developed mechanisms to limit/deprecate user level data via:
- iOS – App Tracking Transparency (ATT): Led to the rate of installs with an IDFA dropping from 80% (due to Limit Ad Tracking) to only 27% (still very important for modelling).
- Android – Google Advertising ID (GAID) deprecation: It is assumed that Google will eventually fully deprecate Android’s user level identifier, but not before 2025.
- Web – 3rd party cookies: After years of planned deprecation of cookies on its Chrome browser, Google decided in July 2024 to keep them after all, and adopt what appears to be a similar approach to ATT. “Instead of deprecating third-party cookies, we would introduce a new experience in Chrome that lets people make an informed choice… We’re discussing this new path with regulators, and will engage with the industry as we roll this out,” the company said in a statement.
Although Google did not provide any details about this “new experience in Chrome that lets people make an informed choice”, it appears that signal loss will be mitigated, going from full to partial deprecation from non-consenting users only. But just how much is impossible to tell as there are still many unknowns: will Google use an opt-out or opt-in mechanism? Single or dual consent (advertiser and publisher side)? Strict/fixed or flexible use of language/UX functionality? Time will tell.
But while the loss of user-level data is currently an iOS reality because of ATT, it will soon expand to Android and later to the Web, affecting all digital marketers. And while each scenario is different, the underlying story remains the same.
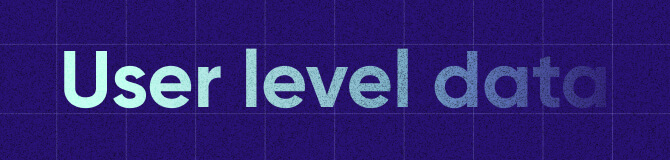
The impact of signal loss on advertisers
While this ecosystem shift is a much-needed step to combat prevalent abuse in user data, it has been a painful adaptation for marketers who rely on user level data for targeting, measurement, and optimization.
1) Measurement
Signal loss has a significant impact on measurement, creating two main challenges for marketers:
a) Fragmented data has been a challenge for years but has increased significantly since iOS14.5 was released. To name just the important data sources:
- MMP attribution (deterministic and probabilistic) and consequent in-app data
- OS and stores (SKAN and later Sandbox) data and consequent in-app data
- SRN attribution data
- iOS consenting user data
- Apple Search Ads data
- Top down measurement data (incrementality and MMM)
- Beyond mobile data: CTV, Web, PC&C, offline
And that’s just in iOS.
How does one reconcile data from so many sources and frameworks to understand performance?
b) Limited and delayed data:
SKAN and AdAttributionKit (SKAN’s successor) offer intentionally delayed signals and only up to 64 values (and only 3 in later postbacks). And despite improvements in AdAttributionKit and Sandbox data, which is said to reach a 30-day LTV, OS data will still come in delayed and limited. As marketers rely on real time, post-install signals for optimization, the fact that OS data is delayed and limited is a significant hurdle.
2) Targeting
When user level data is not available:
- Advertisers cannot create remarketing segments, and as a result they cannot engage effectively with their existing engaged customers, which leads to increased cost per action
- Advertisers cannot create suppression lists so they end up targeting the same users, which leads to increased cost per new acquisition
- Ad networks’ ability to optimize delivery is significantly hurt, which leads to higher costs across the board
In short, signal loss resulting from privacy measures has compromised the completeness of data, posing a risk of misinterpretation and, consequently, misleading insights and the wrong decisions. As a result, a marketer’s job in the signal loss era is 10x harder and so is measurement!
How to overcome signal loss to maintain confidence in measurement
Before ATT, marketers had a rich view with full user level data granularity, like an ultra HD 8k resolution image:
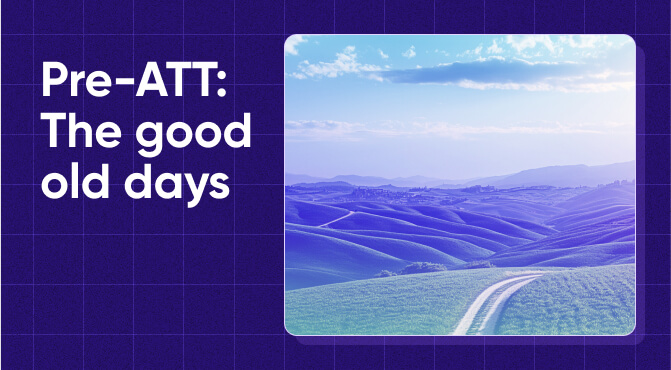
However, the new data reality where user level data is diminishing is a fact and there’s no going back. In this reality, there are a lot of holes, making it difficult to see the full picture.
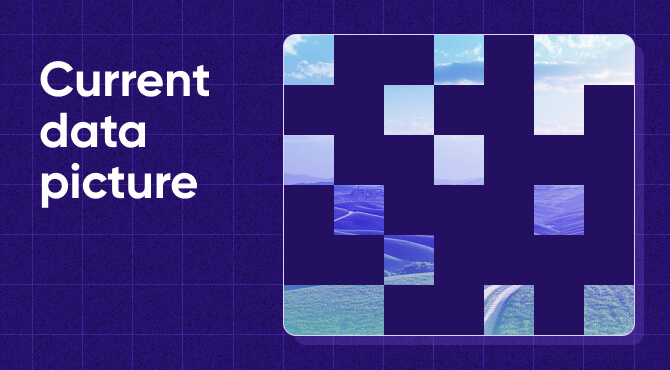
Therefore, marketers must leverage every available data signal and consolidate the different data sources into a single source of truth to measure their campaigns with confidence.
To create a new “data picture”, gaps must be filled wherever possible and new data signals must be added to further compensate. Let’s explore this.
1) Filling in the gaps in the existing data set with modeling
Modeling has been around for years but it wasn’t truly needed in the past since most data was deterministic. However, in a reality of signal loss, it becomes a must, and it can be done with models that do NOT infringe on user privacy. And with AI at our side, the accuracy of models is dramatically improving model accuracy.
Modeling is used to fill in the gaps in:
- OS attribution: Because SKAN data comes in late and limited (and so will data from Sandbox), gaps in the data are created. With modelling, real time signals, geo-level data (not available in SKAN), and LTV data for day 7, day 30, and beyond can be provided.
- Modelling on the media side: SKAN limitations make measurement difficult for Self-Reporting Networks, and since they’ve always relied solely on deterministic attribution, they miss out. By leveraging probabilistic modelling on top of deterministic, they gain lost credit. According to our analysis, Snap increased its share in the iOS install pie by 138%, and its eCPI dropped 46%.
Moreover, large media companies like Google and Meta have also been placing more weight on modeling to navigate and overcome data signal loss challenges in their advertising and marketing strategies.
For instance, Google Ads utilizes machine learning models to refine bidding strategies, while Meta’s Ads Manager platform employs predictive modeling to tailor ad delivery and targeting. Additionally, both companies leverage data modeling to create personalized experiences, reach relevant audiences, and optimize campaign performance.
- Bottom-up (last touch) measurement: Incrementality and Media Mix Modelling (MMM) provide a complementary perspective on campaign performance without having to rely solely on last touch bottom-up signals.
To continue with the high res image analogy, modelling helped fill up some holes, but it’s still not enough.
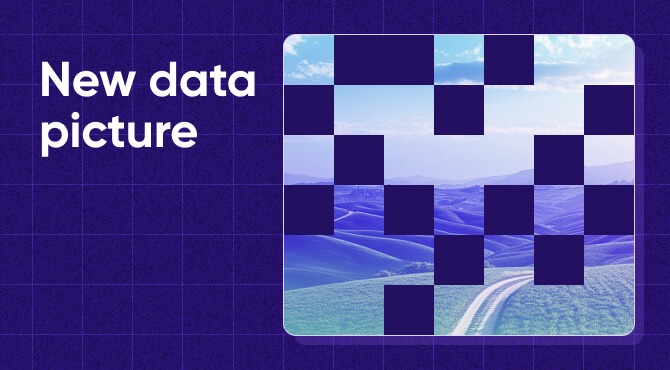
2) Utilizing and creating new signals
- Top of funnel creative and campaign signals: With fewer bottom funnel signals, more focus is now placed on the top of the funnel where there is an abundance of data tied to creatives and campaigns. This covers two areas:
- Data from creatives: Rich and highly granular signals can be obtained, for example, by identifying, within a single ad, scene types (e.g. user generated content, animation/real-life footage, opening/closing) and specific elements (anything from color, scenery, objects, texts etc).
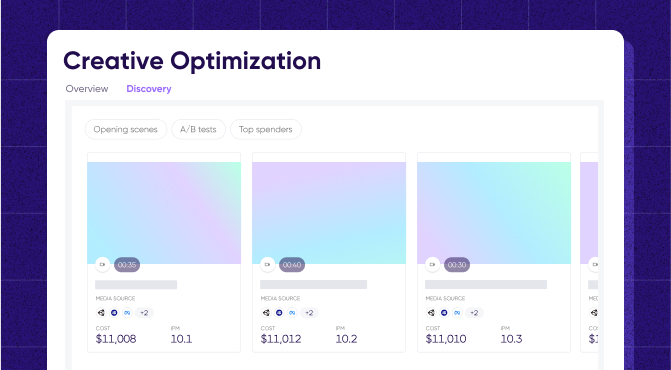
- Enriched-engagement types (EET): The current attribution standard that only looks at clicks or views is flawed. There are many signals in between that can provide valuable performance data and redefine attribution logic.
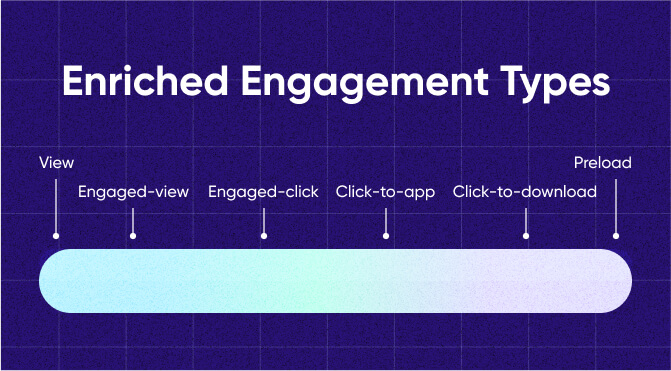
2) [More] 1st party data: With data sharing between different companies limited, the value of 3rd party data sinks. Instead, marketers must step up their use of 1st party data and prioritize its collection and utilization. This includes:
- How to properly collect it, ask users for their info (UX and legal included)
- How to make sure the data is clean and actionable
- How to use it in owned media
- How to use it paid media (remarketing, commerce media)
This data need not remain only within a company’s own environment. A data collaboration platform can then serve as a trusted environment for 1st-party data monetization, audience activation, and measurement. By allowing brands to share this data with different companies in a privacy-compliant way, marketers can truly maximize the potential of their own highly valuable data.
3) Omni-channel signals: Apps, Web, CTV, PC & Console, as well as commerce media networks and out-of-home all present unique opportunities to digitally acquire and engage with a user base. By connecting attribution across channels, marketers can tie new data points and user behavior across their various platforms together to provide a holistic understanding of how cross platform marketing drives business outcomes.
4) Single source of truth (SSOT): As mentioned above, data fragmentation has led to an extremely convoluted reality that’s become largely non-actionable for marketers.
Enter SSOT which creates a single reality by combining data from multiple sources and applies robust tech solutions to identify and remove duplicate installs, fill missing data/gaps in geos, web-to-app, longer LTV, null CV modeling, organic, remarketing etc.
According to our analysis, by consolidating the data into a single view, the average app sees a 29% lift in attributed installs, a 40% drop in eCPI, and a 62% surge in revenue attributed to marketing.
At present, SSOT is centered on iOS but the solution for Web and Android will be similar.
When we add new data signals, we gain information that wasn’t there before for a wider picture of our marketing.
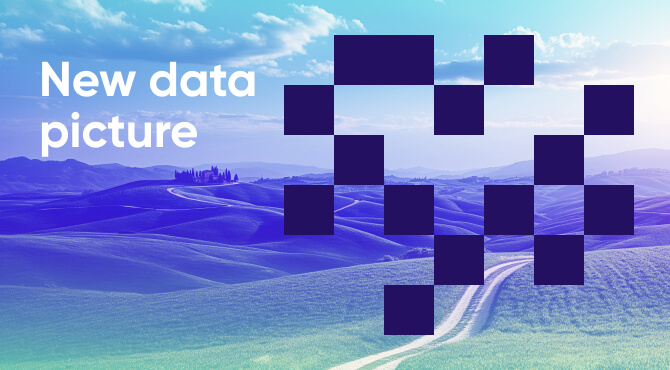
The bottom line
Signal loss has created a challenging environment for performance marketers. With diminishing user level data, driving growth is much harder. Innovative solutions are needed to fill in the gaps in the existing data and create new data signals to allow performance marketers regain control and measure their campaigns with confidence.