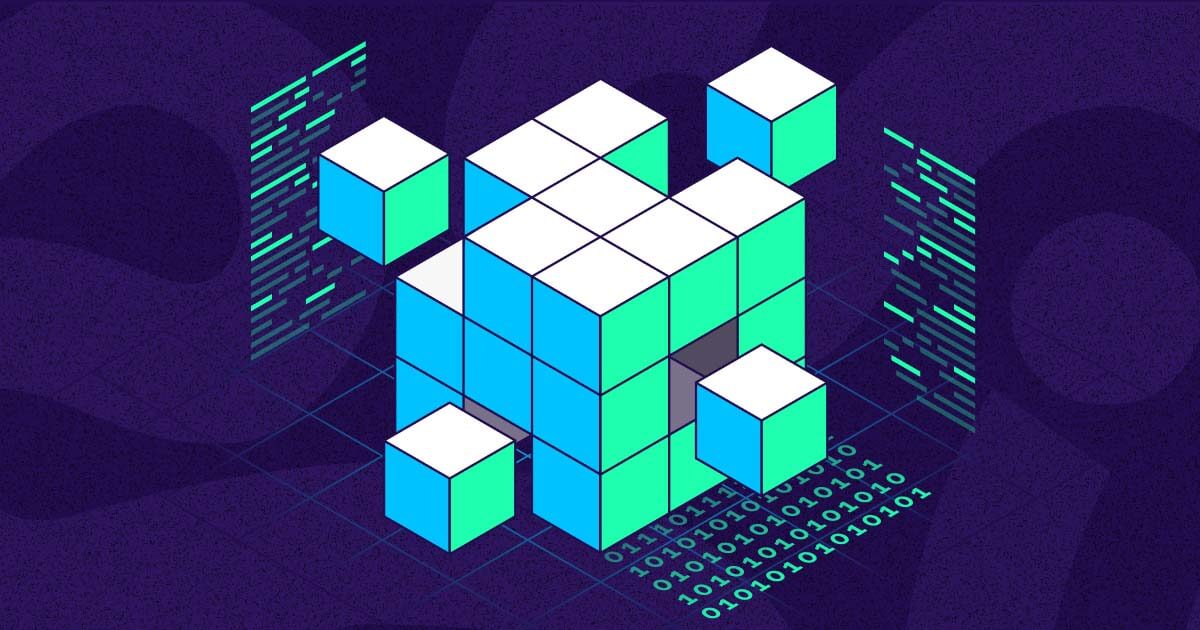
SKAN vs. Sandbox: what advertisers need to know
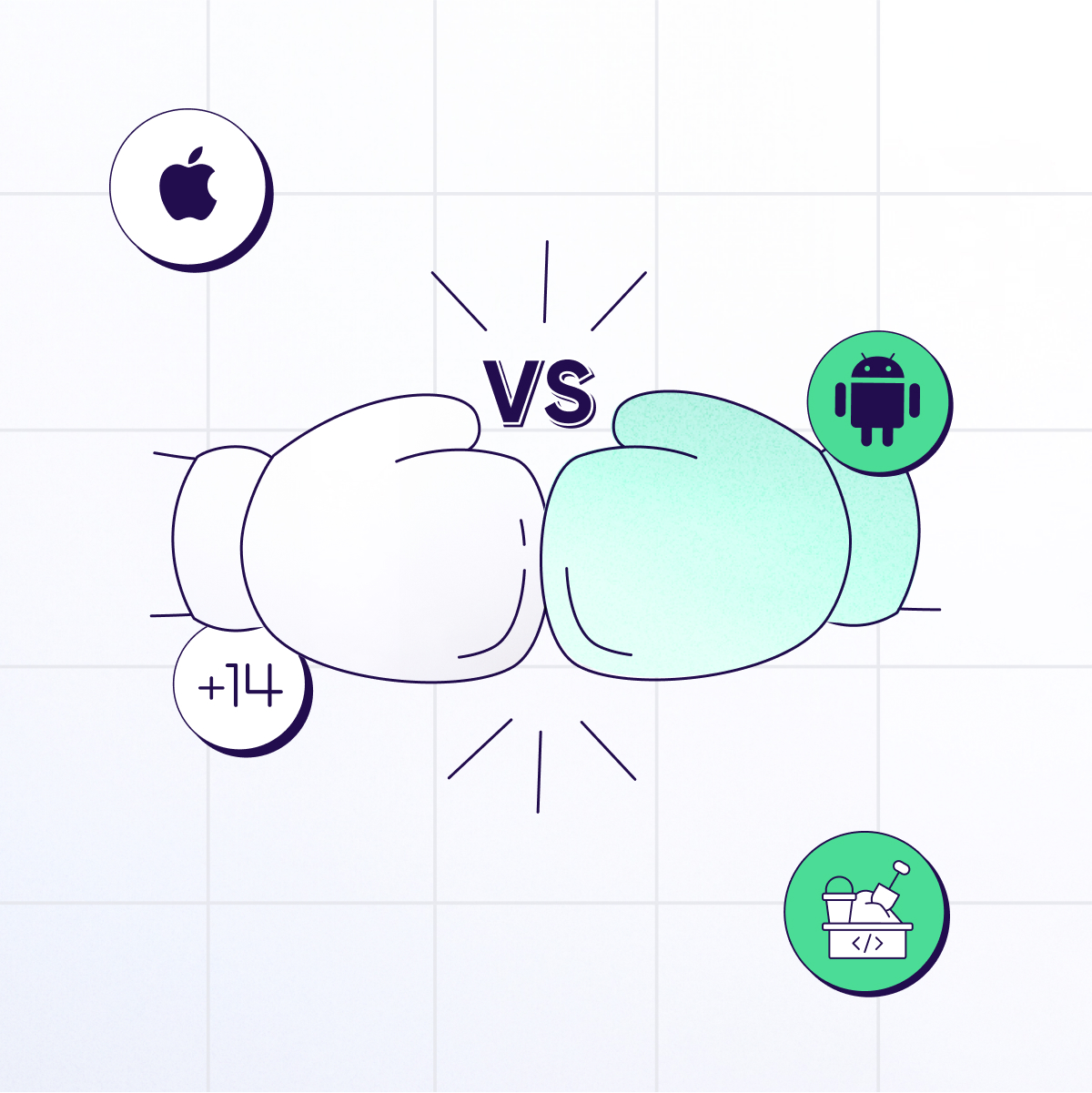
The app marketing world is still adjusting to the evolving privacy changes on iOS, and we are now on the verge of another round of disruptions with Privacy Sandbox on Android and the new opportunities that it introduces.
It’s only natural to stack iOS and Android against each other, so we decided to examine the similarities and differences between SKAN (soon to be revamped as AdAttributionKit) and Privacy Sandbox. Don’t worry though, we’ll (try to) keep things simple.
Before we begin
Google’s Privacy Sandbox is a broad initiative. It’s a suite of marketing infrastructure tools, covering targeting, retargeting, SDKs, and of course attribution.
Since SKAN focuses mostly on attribution, and for the sake of comparing apples to apples (See what I just did there?), we will focus on comparing SKAN to Sandbox’s Attribution Reporting API.
Scope and approach
Doing it the “Apple way”, SKAN is often perceived as a “black box”, naturally specific to iOS only.
With Privacy Sandbox, Google has taken a more collaborative approach and is gradually introducing the different modules for both web (starting with Chrome) and Android, allowing industry leaders such as AppsFlyer to iterate, build innovative solutions using the Sandbox building blocks, provide ongoing feedback, and actively influence the core principles. This approach comes with a cost, as Google often has to reverse decisions – as it did with the recent web cookies deprecation announcement.
Elimination of device IDs
The primary principle for maintaining user privacy is to obscure persistent user identifiers such as IDFAs or GAIDs, and both SKAN and Privacy Sandbox are designed to minimize the use of identifiers.
Notably, Apple’s ATT framework requires user consent to access IDFA, while Google hasn’t announced any plans to use an ATT-style opt-in mechanism.
We’re being asked a lot about the future of GAID (Google Advertising ID) and whether it’s planned to be placed behind an opt-in wall like web cookies are. There’s no formal answer on this one yet. However, bear in mind that none of the Privacy Sandbox APIs use GAID, which can provide a good indication of future direction.
Data aggregation
Both SKAN and Privacy Sandbox use data aggregation, grouping user data into anonymized cohorts to prevent individual profiling. This is a big deal for advertisers, but it’s an even bigger deal for ad networks and publishers that will no longer be able to track users and will have to find new creative ways to optimize their ad performance.
You can expect lots of innovation in this area in the coming months and years.
Reporting
SKAN 4 and AdAttributionKit provide three postbacks and dictate aligning to pre-defined time windows of 2, 7 and 35 days. Kind of like a full-service restaurant that serves meals on specific hours only.
In contrast, the Sandbox Attribution API is like a 30-day buffet. You cannot get wild and eat all that you want due to some “budget” limitations (more on that later), but you’re not tied to strict time windows. When the month ends, it’s time to put the forks down!
While Sandbox’s flexible reporting windows are great news for advertisers, the 30-day limitation might disappoint some marketing managers looking for longer revenue metrics.
Random delays
With privacy-first attribution, data is never received in real-time in order to prevent the option to correlate report data with specific individuals. This forces advertisers to make longer experiments and leads to slower decision-making and reduced agility.
SKAN postbacks arrive with significant delays: 24-48 hours delay for the first postback and 24-144 hours for the 2nd and 3rd postbacks.
Privacy Sandbox incorporates two delay mechanisms to enhance privacy:
Event-level reports (designed for campaign optimization) are delayed by at least 1 day following an ad click and 1-30 days after an ad view—Talk about being late for the party!
Conversely, aggregatable reports (designed for analyzing campaign performance), are available within hours — certainly a relief for advertisers.
Privacy thresholds
There’s a joke about a fan telling a little-known artist, “I bought your album!” and the artist responds, “Oh, it was you!”
This kind of sums up the challenge with privacy-focused ad measurement. When the numbers are small, pinpointing individuals becomes too easy, which isn’t great for privacy.
Both SKAN and Privacy Sandbox address that issue using privacy thresholds, but the implementation is different.
In low volumes, SKAN simply doesn’t give you information on post-install activity (as well as campaign information). That’s the main reason why we at AppsFlyer developed a null modeling solution for SKAN to compensate for that signal loss. Privacy Sandbox uses the notion of “noise”. It adds fake data to mask the real numbers. The noise can be significantly deceiving when data volumes are low, but as volume grows the relative noise size reduces and becomes negligible.
Tip: For both SKAN and Sandbox, low data volumes can pose challenges for advertisers. It’s best to allocate adequate budgets to each media source and avoid splitting campaigns excessively. This strategy ensures data volumes are sufficient to bypass privacy thresholds, providing more reliable reports. The more focused your budget, the more reliable your data.
Bit-based attribution
Both SKAN and Sandbox use “bits” to represent conversion or campaign data. Values represented by bits are more challenging to use but they ensure data is controlled, standardized, and more importantly: no direct identifiable information (like names, IDs, or even specific actions) is transmitted.
SKAN uses postbacks to share conversion data with advertisers and ad networks. Privacy Sandbox provides APIs available for ad tech companies to access the attribution reports.
To help you get the most out of those bits, MMPs like AppsFlyer offer standardized methods for converting and interpreting bits into human-friendly metrics and actionable insights.
Data granularity
SKAN allocates 2-4 digits for source identifiers (depending on the number of installs) and permits only six bits for post-install conversion data (equivalent to 64 possible conversion values). This significantly limits the granularity with which marketers can analyze campaign performance and post-install activities.
In contrast, Privacy Sandbox offers 128 bits for the campaign sources and post-install events. I’ll save you the calculations and the weird numbers but trust me, you’ll get over a billion times more data with Sandbox than you do with SKAN.
Keep in mind, though, that in the Privacy Sandbox each source event, like an ad click, has a maximum number of ‘values’ it can use, known as the contribution budget. Sandbox limits you to certain contribution budgets and adds random noise. This means that even though the data can be pretty granular, you’ll need to plan your budgets carefully and prioritize upfront.
Technical complexity
SKAN is like a black box managed by Apple, delivering just the essentials like winning campaigns and conversion counts.
In contrast, Privacy Sandbox is designed as a set of building blocks—enabling industry players like AppsFlyer to build their own solutions on top of it. These solutions must comply with strict cloud infrastructure guidelines to maintain privacy.
In addition, Sandbox offers a cross-network last-click attribution through the presence of a neutral player such as an MMP. That means AppsFlyer and other MMPs will have a significant role in the Sandbox attribution data flow providing insights on cross-network last-click attribution.
This key difference makes the Sandbox a more complex technology but also opens up opportunities for innovation and disruption in the industry.
Presenting the full picture
Both SKAN and Privacy Sandbox are tailored to their respective operating systems (iOS and Android), making it hard for advertisers to see the full picture.
Add the fact that some ad networks haven’t fully adopted SKAN yet (which also doesn’t support Apple Search Ads), along with the fact that Privacy Sandbox is just getting started, and you’re left with a reality split across several ‘buckets of truth’—far from ideal for analyzing global campaign performance and make informed decisions.
In such a fragmented landscape, having a single source of truth is crucial. AppsFlyer continues to bridge this gap with its pioneering Single Source of Truth (SSOT), which consolidates all campaign data across media sources and platforms into one centralized view.
Summary
In a privacy-first era, advertisers need to adapt to a new reality in which personal information is never revealed, measurement is aggregated and anonymous, the reporting intervals are long, and data is delayed and obscured.
While SKAN keeps attribution simple but very limited, Privacy Sandbox offers a much wider range of capabilities and data granularity, but requires a much heavier technological effort and the creation of complementary solutions.
AppsFlyer is already developing its next-generation products that will support Privacy Sandbox, enabling advertisers to seamlessly adopt these new technologies, protect user privacy, and enhance their marketing strategies.